A brain implant that can predict what you are trying to say
A new brain implant developed at Duke University might help patients with speech disabilities communicate again.
Developed by a team of neuroscientists, neurosurgeons and biomedical engineers at the university, the implant can translate a person’s brain signals into speech through a brain-computer interface. It is significantly more efficient at decoding brain signals and boasts a much higher resolution than the current generation of prosthetics, and seems set to evolve into the medical miracle that scores of patients who have lost their voice—such as those with debilitating neurological conditions like amyotrophic lateral sclerosis—are waiting for.
“The current tools available to allow them to communicate are slow or cumbersome,” noted Professor Gregory Cogan from the university’s School of Medicine, who is one of the lead researchers of the project. These prosthetics decode brain signals at just about half the speed of natural speech, which is akin to listening to an audiobook at half-speed. This limitation is in part due to the design, which relies on a paper-thin film with activity sensors to measure brain signals. Most of these films are large and can only accommodate so many sensors, up to 128, which limits the amount of information that can be decoded.
“We did not want to add any extra time to the operating procedure, so we had to be in and out within 15 minutes. As soon as the surgeon and the medical team said ‘Go!’ we rushed into action and the patient performed the task.”
To overcome this limitation, Cogan teamed up with Associate Professor Jonathan Viventi from the department of biomedical engineering who specialises in making high-density, ultra-thin, and flexible brain sensors. To improve the decoding rate of the new implant, Viventi and his team packed an impressive 256 microscopic sensors onto a postage stamp-sized piece of flexible, medical-grade plastic. Since neurons that are just a grain of sand apart can have wildly different activity patterns when coordinating speech, such high-density packing of sensors helps to distinguish signals from neighbouring brain cells and improves the accuracy of their translation into speech.
In the lab, PhD candidate Kumar Duraivel analyses a colourful array of brain-wave data. Each unique hue and line represent the activity from one of 256 sensors, all recorded in real-time from a patient's brain in the operating room // Credit: Dan Vahaba, Duke University
Once the implant was fabricated, the next big task was to test it in patients. For this, Cogan and Viventi collaborated with a team of neurosurgeons from Duke University Hospital, who recruited four patients to test the implants. The researchers temporarily placed the implants on the surface of the brain of these patients who were undergoing awake brain surgeries for other conditions, who then had to perform a simple listen-and-repeat task: hearing a series of meaningless words like “ava”, “kug” and “vip”, and saying them out loud. The implant recorded the brain signals generated in patients’ speech motor cortex during the task. In the intense setting of an operation theatre where time is a crucial element, the entire activity was performed in 15 minutes.
“We did not want to add any extra time to the operating procedure, so we had to be in and out within 15 minutes. As soon as the surgeon and the medical team said ‘Go!’ we rushed into action and the patient performed the task,” said Cogan.
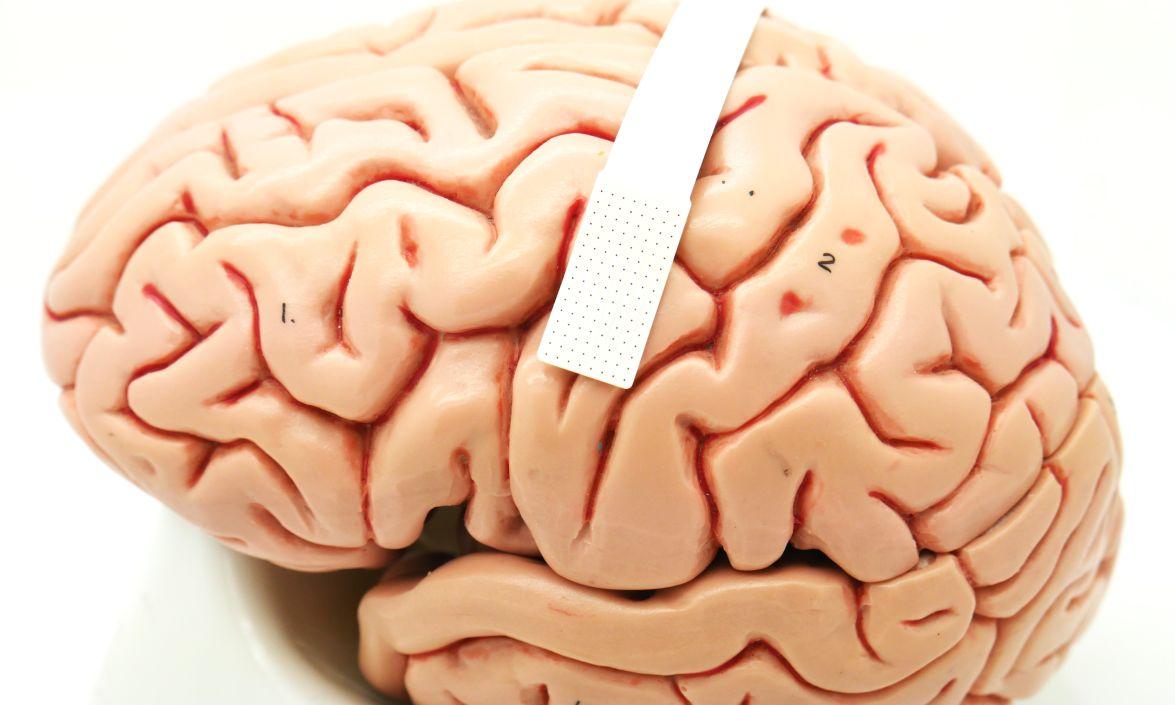
A device no bigger than a postage stamp (dotted portion within white band) packs 128 microscopic sensors that can translate brain cell activity into what someone intends to say // Credit: Dan Vahaba, Duke University
Following this, Suseendrakumar Duraivel, a graduate student at the biomedical engineering lab and the first author of the study, fed brain signals from each patient into a machine learning algorithm and compared the sound predictions it made with actual speech data.
Duraivel found that the algorithm predicted the first sound of the three-alphabet words (eg., /g/ in ‘gak’) with 84 per cent accuracy, but was less accurate in predicting the second and third sounds (eg., /g/ in kug). It also struggled to differentiate between similar sounds (eg., /p/ and /b/). The algorithm had an overall accuracy of 40 per cent—a remarkable technical feat considering that this was achieved with just 90 seconds of spoken data from the 15-minute test, compared to standard tools that require hours or days of data to achieve similar values.
The promising findings have attracted US$2.4 million in funding from the National Institutes of Health, and the research team plans to use this money to improve the implants into cordless devices.
“We’re now developing the same kind of recording devices, but without any wires,” enthused Cogan. “You’d be able to move around, and you wouldn’t have to be tied to an electrical outlet, which is really exciting.” Along with this, bringing the decoding algorithms up to speed will be the primary focus for the research team before they can chart out a ‘bench-to-bedside’ translation pathway for the implants.